Introduction
The approach to sports games involves analysing performance parameters to optimise decision-making by players and coaches. Game analysis is the tool used to understand critical periods of the game’s phases during training and competition [1-4]. In this context, this tool enables the analysis and interpretation of teams during different moments and phases of games, identifying factors sensitive to team performance [5, 6]. Therefore, as sports evolve in offensive and defensive strategies, it becomes necessary to obtain information about game patterns so that decision-making can be adjusted to the demands of the teams [7-9]. When considering volleyball, the subject of this research, it was observed that games can be divided into functional phases known as game complexes (K’s) [5, 10, 11]. Based on traditional and fundamental knowledge of this sport, Complex I is identified as comprising reception, setting, and attack and Complex II includes serve, block, defence, and counter-attack [10]. However, with the need to better understand all phases of the game and the construction of the attack from different offensive structuring conditions, increasing the understanding of offensive constructions in counter-attacks, the game was sequenced into the following complexes: (a) Complex 0 (K0): consisting of the serve; (b) Complex I (KI): reception, setting, and attack; (c) Complex II (KII): composed of the counter-attack following the defence of the opponent’s attack; (d) Complex III (KIII): defined as the counter-attack following the opponent’s counter-attack; (e) Complex IV (KIV): counter-attack following the attack coverage; (f) Complex V (KV): counter-attack following freeballs [5, 11].
Understanding volleyball through game complexes allows for the identification of team behaviour patterns according to the start of the offensive construction (e.g., from reception or defence), as well as according to the game conditions that may occur in the system, that is, in ideal conditions for offensive construction, or out system with limited conditions for offensive construction [5, 12]. In this context, research on men’s and women’s volleyball has shown that men’s teams score more attack points after reception (KI) [8, 13], likely due to greater ball control that occurs after reception, allowing for better offensive structuring [4, 14, 15]. On the other hand, in women’s volleyball, a sustaining game is observed, characterised by more attacks after defences, suggesting that slower sets and less powerful attacks in the offensive phase allow for better defensive structuring and a higher occurrence of counter-attacks [16, 17].
Game analysis in volleyball is mainly conducted through the analysis of game actions, considering a specific play complex [6, 15, 18], complicating the game’s understanding. In this context, there is limited information about the transitions between complexes that occur more frequently to support training in the sport according to the level of competition [11, 12, 19]. Recent research in women’s volleyball has used Social Network Analysis (SNA) to provide a relative understanding of the importance and behaviour among game complexes [20, 21], has shown that K0 was the most frequent, followed by KI from receptions that allowed organised attacks, and KII which did not allow continuity of play [5, 10]. When considering Spanish women’s volleyball, sequences such as K0-KI-KII, K0-KI-KIV, and K0-KI-KV are more likely to occur [10], whereas in Brazilian volleyball, K0-KI, K0-KI-KII are more common [5]. Despite this research on game complexes in women’s volleyball, it is necessary to consider the sample size, as only seven games were analysed in both studies, suggesting that the small samples indicate a specific game pattern to a limited number of volleyball teams.
Given the above, recognising differences in the type of game played between men and women and the lack of studies on women’s volleyball, it is important to identify occurrence patterns that specify the type of game played in women’s volleyball and each set. This enables the planning of training sessions that are adjusted to the reality of the game, as it is not possible to extrapolate research results from men’s volleyball to women’s due to considerable differences in the type of game played [16, 17]. Therefore, this study aimed to analyse the game flow in high-level women’s volleyball by sequencing game complexes per set. The hypotheses of the present study were: I - the eigenvector centrality values will be higher in Complexes 0, I, and II, regardless of the set played; II - the sequences of K0 with continuity effect and KI with point effect or K0 with continuity effect, KI with continuity effect, and KII with point or error effect will be the most recurrent, regardless of the set played; and III - there will be no difference in the number of game complexes required to obtain the point between sets.
Material and methods
Sample
This study’s robustness and differential included analysis of all the teams that participated in the 2021/2022 Brazilian Women’s Superliga, totalling 135 out of the 148 matches played (13 matches were excluded from the sample due to a lack of access to the games). Between 14 and 25 matches were analysed for each team. The Brazilian Women’s Volleyball Superliga was selected for analysis due to its relevance, as Brazil ranks among the top 3 teams in the world [22].
Data collection
All matches were filmed from above the court using a Sony® high-definition camera (1080p) positioned approximately 7-9 m behind the end line of the court and five metres above ground level. To analyse the games, a physical education professional with over ten years of experience in volleyball performance analysis trained one master’s student and two undergraduate students. All had experience as volleyball athletes. For reliability testing, 15 matches were reanalysed, exceeding the 10% reference value [23]. Cohen’s Kappa values for intra-observer reliability ranged from 0.90 to 0.99, with standard errors of 0.03 and 0.01, respectively. For inter-observer analysis, the values ranged from 0.95 to 1, with a standard error of 0.02. These values are above the recommended threshold of 0.75 [24].
Variables
Set played: The set played was considered, with the first four sets ending when a team reaches 25 points, provided there is at least a two-point difference from the opposing team; otherwise, the set ends after the 25th point with a two-point difference. The fifth set ends when a team reaches 15 points, requiring at least a two- point difference from the opposing team; otherwise, the set ends after the 15th point with a two-point difference. Game complex: For the analysis of game complexes, K0, KI, KII, KIII, KIV, and KV were considered [5, 10]. In KV, freeballs and downballs were included due to their similarities in offensive construction and reduced occurrences. The flow and possible sequences of game complexes are illustrated in Figure 1.
Complex effect: This variable considered the effect of the attack at the end of each play complex, classifying it as an error (0), attack block (1), continuity (2), or point (3) [25, 26]. The sequence of game complexes within each rally and the attack’s effect was recorded. It is noted that serving actions were classified similarly to attacks, except for blocking, as by rule, serves cannot be blocked in volleyball.
Statistical analysis
The data were recorded in a Microsoft Excel 2020 spreadsheet for data quality control. The SNA was conducted using Gephi 0.8.2-beta software for Mac (version 10.10.3, France). SNA was employed because this statistical procedure considers the connectivity and specificity of relationships among all contextual variables, providing a comprehensive view of the type of game played. For data analysis, SNA was used to create a network that related all sequences of game complexes by set, considering undirected nodes, allowing observation of how game complexes connect based on their effects. Eigenvector centrality was utilised, as it reflects the relationship among game complexes, based on the understanding that a node has higher centrality when it is connected to more central nodes, with a centrality value ranging from 0 to 1 [27, 28]. Therefore, node centrality depends on adjacent nodes and their interaction characteristics [19]. To visually highlight the magnitude of each node’s eigenvector values, the font size of each node was adjusted accordingly. The graph was organised using the Fruchterman-Reingold layout (area 100000) [29].
In addition to eigenvector analysis, inferential analysis was performed using the chi-square test with Monte Carlo correction when less than 20% of the cells had values less than 5, to verify the association between study variables according to the set played. Residual adjustments were calculated to identify which cells showed statistical significance in explaining the relationship between two variables. In data treatment, the significance level was set at 5% (p < 0.05), and the effect size was calculated using phi (φ) (small: V < 0.1, moderate: 0.1 < V < 0.3, large: V > 0.3). The data were analysed using SPSS (Statistical Package for the Social Sciences) version 29.0 for MacOS.
Results
The SNA resulted in a graph identifying the connections between the game complexes and their effects per set (Figure 2). Table 1 presents the eigenvector values.
Figure 2
Graph of complex sequencing based on eigenvector values. Each node displays in its name the set, the complex, and the effect that each one represents. Thus, “2 set K3-0” indicates that K3 is associated with the effect of continuity in the second set
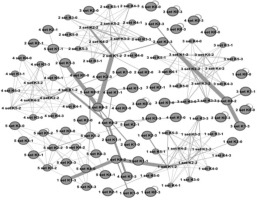
Table 1
Eigenvector values for game complexes per set 1st set
As observed in Table 1 and Figure 2, the highest eigenvector values were associated with the effect of continuity, except for K0. In the first four sets, the highest eigenvector values were for Complexes III, IV, and V for the continuity effect. In the fifth set, the highest eigenvector values were similar among Complexes II, III, IV, and V for the continuity effect.
Considering the association between sequencing and the set played, 608 different sequencing types were observed. The results showed no association between the sequencing of game complexes and the set played (χ2 = 2470.01, φ = 0.34, p = 0.272). The most recurrent sequences were: K0-2-KI-3 (33.8%), K0-0 (10.3%), K0-2-KI-0 (7.1%), K0-2-KI-2-KII-3 (7.1%), K0-2-KI-1 (6.9%), and K0-3 (4.5%). The analysis of the complexes showed no association between the number of complexes and the set played (£ = 17.63, φ = 0.03, p = 0.346), as shown in Table 2.
Table 2
Association between the set played and the number of Ks
Discussion
The present study aimed to analyse the flow of play in high-level women’s volleyball by sequencing game complexes by set. We listed three different hypotheses for the study: I - the eigenvector centrality values will be higher in Complexes 0, I, and II, regardless of the set played; II - the sequences of K0 with continuity effect and KI with point effect or K0 with continuity effect, KI with continuity effect, and KII with point or error effect will be the most recurrent, regardless of the set played; and III - there will be no difference in the number of game complexes required to obtain the point between sets.
The first hypothesis was refuted. Network analysis showed that the continuity effect presented the highest eigenvector value across all game complexes and sets analysed, with the highest eigenvector values occurring for Complexes II and III up to the third set. In the fourth set, the highest eigenvector values were observed for Complexes III, IV, and V, and in the fifth set, the highest eigenvector values were for Complexes II, III, IV, and V for the continuity effect. These results are consistent with the literature, which demonstrated higher eigenvector values for the continuity effect in women’s volleyball game complexes, where points are associated with Complexes II, III, and IV [5, 10].
Although no studies specifically analysed game complexes per set, the higher eigenvector values for Complexes III, IV, and V in the fourth and fifth sets might be explained by mental fatigue caused by the demands of the game. Mental fatigue is characterised by a sense of tiredness and/or lack of energy after long periods of cognitive activity [30], which impairs decision-making and athletic performance [31-35]. In this context, considering that there is a sustaining game in women’s volleyball due to lower power in terminal actions such as serving and attacking [26, 36], as well as a slower-paced game [26, 36, 37], it can be considered that the fatigue accumulated throughout the match can lead to poorer decision-making, consequently altering the sequence of game complexes, as indicated by the results found in this study.
The second hypothesis was refuted. Contradicting these results, a previous study analysed two matches (six sets) from the 2015 Copa de la Reina and five matches from the same competition in 2016, observing that the most frequent sequences were: K0-KI-KII, followed by K0-KI-KIV, K0-KI-KV, and K0-KI-UK [10]. They also found that Complexes II, III, and IV were more closely associated with non-continuity. In another study, Rocha et al. [6] analysed seven matches from the Women’s Brazil Cup 2020, showing that 77% of the sample had between one and four-game complexes (Table 1), suggesting that high-level women’s volleyball involves more continuity actions, with positive associations between KI, KII, KIII, and KV and points. In this context, the differences observed between the findings of this study and existing literature may be due to the number of games analysed, further highlighting the differences between all the teams participating in the competition and the analysis of matches between teams with different performance levels.
The third hypothesis was confirmed. In this regard, Hileno et al. [10] observed that nearly half of the points were scored after three complexes like K0-KI-KII, K0-KI-KIV, and K0-KI-KV. In another study, Laporta et al. [5] found that sequences with two or three com plexes represented 65% of the sample, suggesting that women’s volleyball may move toward game patterns observed in men’s volleyball. Despite the limited number of studies on women’s volleyball and game sequencing, the results indicate a sustaining game and continuity in play. Additionally, as discussed in the first hypothesis, it must be considered that as the number of sets increases during a match, there is greater mental fatigue [30], which reverberates in poorer athletic performance [31-35]. This, in turn, can modify the sequencing of game complexes without altering the number of complexes required to score a point.
Overall, the results show that in women’s volleyball, there is an emphasis on avoiding errors at the expense of scoring points, indicating a difference in strategy compared to men’s volleyball, where athletes take the risk of errors by prioritising faster sets and powerful attacks [3, 6, 38]. Likely, the type of game played is based on physical characteristics, precisely strength and power, which confer greater continuity to the game in women’s volleyball [26, 36, 37]. In this regard, it should be noted that the present study analysed 90% of the matches from the 2020-2021 Women’s Superliga, considering sequencing from matches between higher and lower-ranked teams. Therefore, the number of sets, type of matchup, and stage of the championship may influence the type of game played [18, 39, 40], with distinct sequencing patterns potentially arising from the specificities of the matchup.
However, it must be acknowledged that this study, like any other, has limitations. Although many matches were analysed, the confrontations between teams of different performance levels were not considered, which may reveal different sequencing patterns according to the opponent’s performance level, or the players’ mental fatigue, other types of psychological pressures throughout the sets, or varying strategies employed by the coaches. In this context, this research provides volleyball coaches with an understanding of the importance of training in attack or counter-attack situations from various scenarios, as sustaining play is a key aspect of offensive strategies in women’s volleyball. Therefore, the training of women’s teams should consider that initiating offensive construction can alter the course of game sequencing, making it necessary to consider offensive construction from less frequent conditions. For example, it may be advantageous for an attack to be deliberately directed against the block, allowing the team to rebuild the offensive phase starting from KIV.
Conclusions
Women’s volleyball features game strategies oriented towards sustaining play, promoting game continuity regardless of the set played. Additionally, the number of game complexes required to score a point ranges between two and three complexes, with over 600 distinct sequences observed in the sample analysed in this manuscript. Therefore, coaches should consider the dynamics in women’s volleyball matches, preparing teams for less risky and more continuous play.